[Title]
Abdominal Aortic Thrombus Segmentation in Postoperative Computed Tomography Angiography Images using Bi-directional Convolutional Long Short-term Memory Architecture
[Authors]
Byunghoon Hwang, Hyoseok Hwang (Corresponding Author)
– Department of Software Convergence, Kyung Hee University
Jihu Kim, Sungmin Lee, Suhyeon Kim, Younhyun Jung
– Department of Software, Gachon University
Eunyoung Kim, Jeongho Kim
– Department of Radiology, Gachon University Gil Medical Center,
[Abstract]
Abdominal aortic aneurysm (AAA) is a fatal clinical condition with high mortality. Computed tomography angiography (CTA) imaging is a preferred minimally-invasive modality for long-term postoperative observation of AAA. Accurate segmentation of the thrombus region of interest (ROI) in a postoperative CTA image volume is essential for quantitative assessment and rapid clinical decision making by clinicians. Few investigators proposed the adoption of convolutional neural networks (CNN). Although these methods demonstrated the potential of CNN architectures by automating the thrombus ROI segmentation, the segmentation performance can be further improved. The existing methods performed the segmentation process independently per 2D image and were incapable of using adjacent images, which could be useful for the robust segmentation of thrombus ROIs. In this work, we propose a thrombus ROI segmentation method to utilize not only the spatial features of a target image but also the volumetric coherence available from adjacent images. We newly adopted a recurrent neural network, bi-directional convolutional long short-term memory (Bi-CLSTM) architecture, which can learn coherence between a sequence of data. This coherence learning capability can be useful for challenging situations, for example, when the target image exhibits inherent postoperative artifacts and noises, the inclusion of adjacent images would facilitate learning more robust features for thrombus ROI segmentation. We demonstrate the segmentation capability of our Bi-CLSTM-based method with a comparison of the existing 2D-based thrombus ROI segmentation counterpart as well as other established 2D- and 3D-based alternatives. Our comparison is based on a large-scale clinical dataset of 60 patient studies (i.e., 60 CTA image volumes). The results suggest the superior segmentation performance of our Bi–CLSTM-based method by achieving the highest scores of the evaluation metrics, e.g., our Bi-CLSTM results were 0.0331 higher on total overlap and 0.0331 lower on false negative when compared to 2D U-net++ as the second-best.
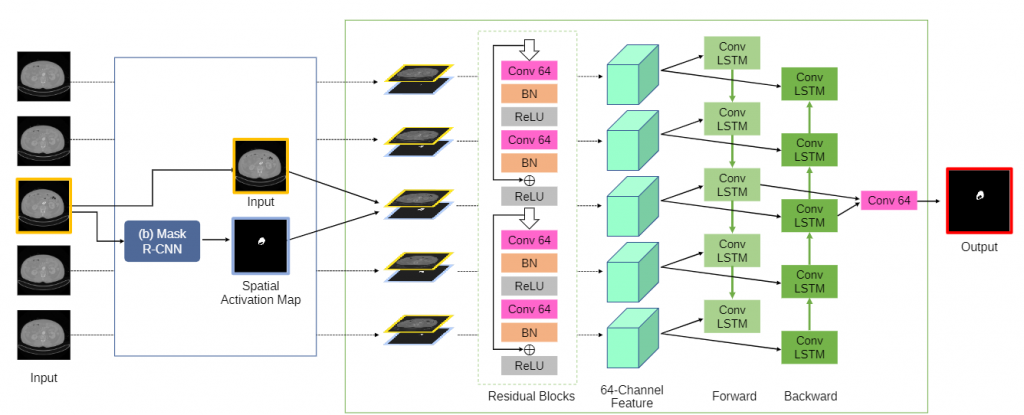
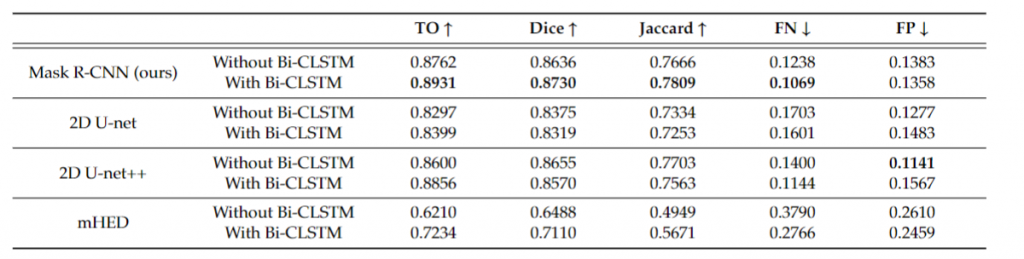