[Title]
Real-Time Automated Solubility Screening Method Using Deep Neural Networks with Handcrafted Features
[Authors]
Minwoo Jeon, Geunhyeok Yu, Hyoseok Hwang
– Department of Software Convergence, Kyung Hee University
Hyundo Choi, Gahee Kim
– Material Research Center, Samsung Advanced Institute of Technology, Samsung Electronics
[Abstract]
Solubility measurements are essential in various research and industrial fields. With the
automation of processes, the importance of automatic and real-time solubility measurements has
increased. Although end-to-end learning methods are commonly used for classification tasks, the
use of handcrafted features is still important for specific tasks with the limited labeled images of
solutions used in industrial settings. In this study, we propose a method that uses computer vision
algorithms to extract nine handcrafted features from images and train a DNN-based classifier to
automatically classify solutions based on their dissolution states. To validate the proposed method, a dataset was constructed using various solution images ranging from undissolved solutes in the form of fine particles to those completely covering the solution. Using the proposed method, the solubility status can be automatically screened in real time by using a display and camera on a tablet or mobile phone. Therefore, by combining an automatic solubility changing system with the proposed method, a fully automated process could be achieved without human intervention
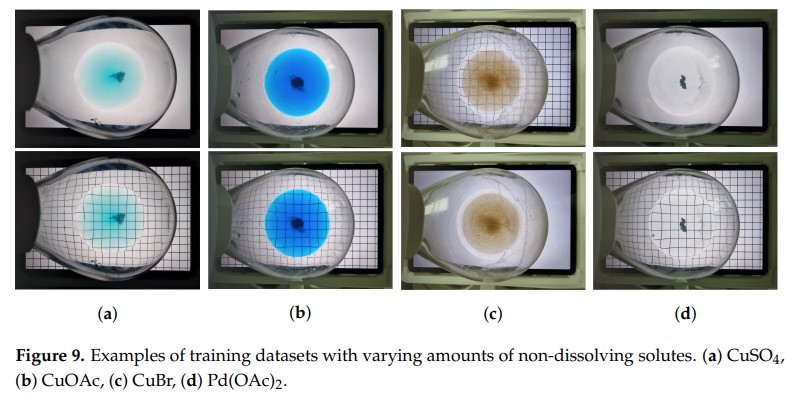
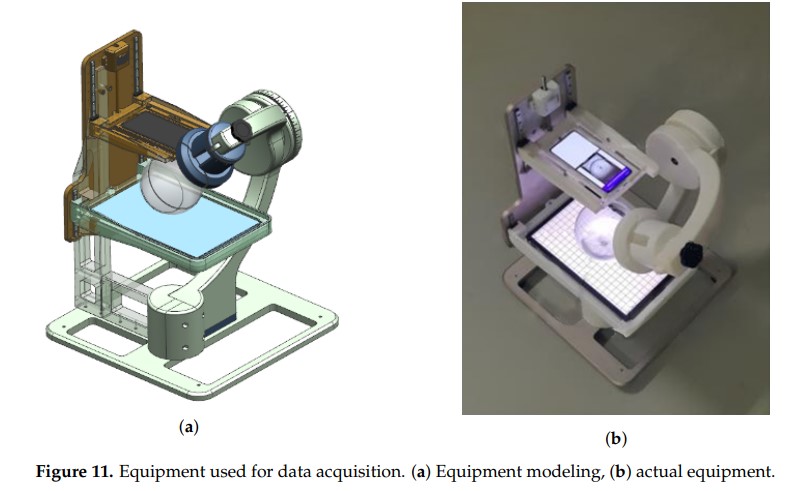